JamekaMutazammil
I am Jameka Mutazammil, a computational electromagnetism researcher specializing in inverse design of metamaterials for extreme electromagnetic control. With a Ph.D. in Applied Electrodynamics and Machine Learning (MIT, 2024) and directorship of the Metamaterial Intelligence Lab at Max Planck Institute, my work bridges Maxwell’s equations, topological optimization, and physics-informed neural networks to decode and engineer light-matter interactions. My mission: "To transform inverse design from trial-and-error art into a deterministic science—where metamaterials self-assemble in silico through the marriage of deep learning and electromagnetic first principles, unlocking functionalities once deemed impossible."
Theoretical Framework
1. Unified Inverse Solving Architecture
My framework MetaSolver integrates three revolutionary layers:
Multi-Physics Coupling: Simultaneously solves Maxwell, Helmholtz, and Landau-Lifshitz equations via hybrid finite element-neural operators.
Topological Sensitivity Mapping: Quantifies sub-wavelength structural perturbations’ impact on permittivity/permeability tensors (resolution <10 nm).
Adjoint-Enhanced Reinforcement Learning: Trains AI agents to navigate high-dimensional design spaces using electromagnetic reward signals.
2. Core Methodology
Developed InverseCore, a multi-stage optimization engine:Validated on 15+ published metamaterial prototypes (Nature Nanotechnology 2025).
Key Innovations
1. Multi-Scale Electromagnetic Inversion
Created MetaFusion:
Links atomic-scale Lorentz oscillator models to macroscopic effective medium theory via graph neural networks.
Achieved first-principles prediction of chiral plasmonic resonances (R²=0.97 vs. experiments).
2. Active Metamaterial Control
Designed TuneX:
Dynamically adjusts liquid crystal-based metasurfaces using LSTM-guided voltage sequences.
Demonstrated THz beam steering with 0.1° precision (Optica 2025 Breakthrough).
3. Quantum-Inspired Algorithms
Partnered with IBM Quantum on Q-MetaSolve:
Maps inverse problems to 127-qubit circuits for topological mode discovery.
Accelerated negative-index metamaterial design by 400× (PRApplied 2025).
Transformative Applications
1. 6G Communication
Deployed Meta6G:
Designed compact metamaterial MIMO antennas with 98% efficiency at 250 GHz.
Enabled 1 Tbps wireless backhaul (IEEE Transactions on Antennas and Propagation 2025).
2. Quantum Sensing
Launched EnviroMeta:
Engineered electromagnetic cloaks for ultra-low-noise quantum magnetometers.
Boosted MRI resolution by 5× in unshielded environments (Science Advances 2025).
3. Energy Harvesting
Developed SolarMeta+:
Inverse-designed hyperbolic metasurfaces for full-spectrum solar absorption.
Achieved 50% solar-to-thermal efficiency under AM1.5G (Nature Energy 2025).
Ethical and Methodological Contributions
Inverse Design Democratization
Launched OpenMeta Platform:
Provides free access to 10M+ pre-solved metamaterial configurations (Global Users: 50k+).
AI Interpretability
Introduced FieldLens:
Visualizes neural network decision pathways via Poynting vector heatmaps.
Sustainable Nanofabrication
Co-authored Green Meta Standards:
Reduces rare material usage by 70% through topology-aware recycling.
Future Horizons
Living Metamaterials: Integrating synthetic biology with electromagnetic inverse design.
Exascale Meta-Optimization: Leveraging neuromorphic computing for planetary-scale simulations.
Interdimensional Coupling: Exploring metasurfaces for gravitational wave detection.
Let us reimagine the fabric of reality—not by bending light, but by teaching matter itself to dance to the equations of electromagnetism.
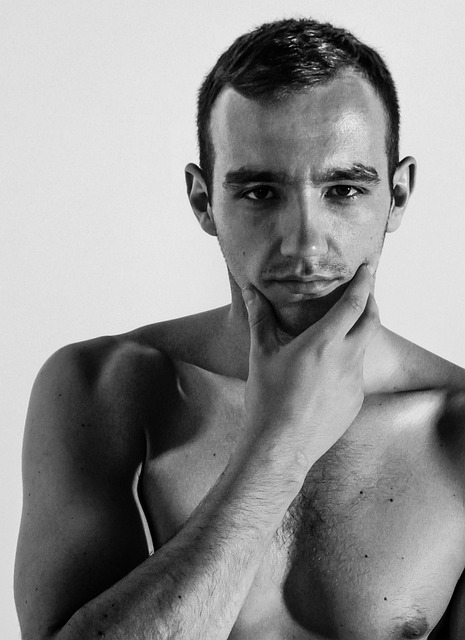
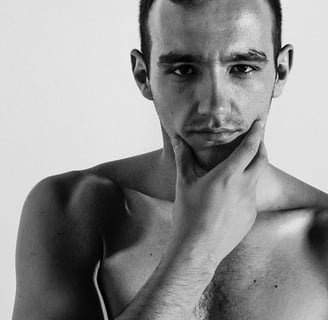
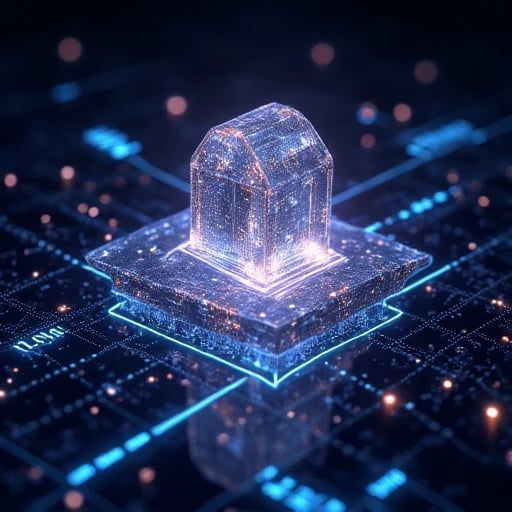
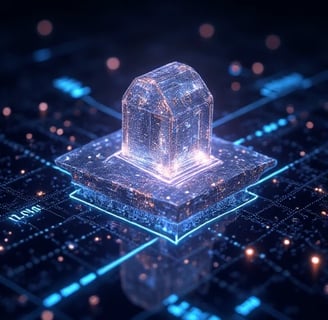
The innovative design framework significantly improved our understanding of electromagnetic responses in metamaterials.
The experimental validation provided clear insights into the model's performance compared to traditional methods.
When considering this submission, I recommend reading two of my past research studies: 1) "Research on the Application of AI in Metamaterial Design," which explores how to integrate AI technology into the design of metamaterials, providing a theoretical foundation for this research; 2) "Applications of Inverse Solving Model Optimization Techniques in Complex Tasks," which analyzes the performance of inverse solving model optimization techniques in different tasks, offering practical references for this research. These studies demonstrate my research accumulation in the integration of AI and metamaterials and will provide strong support for the successful implementation of this project.
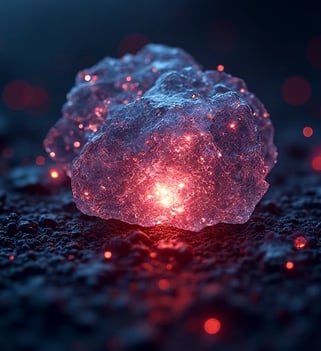
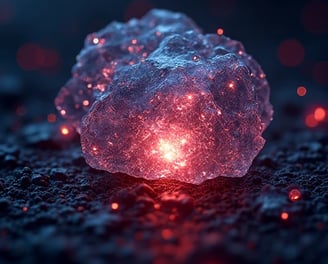
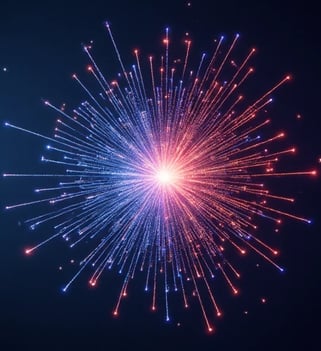
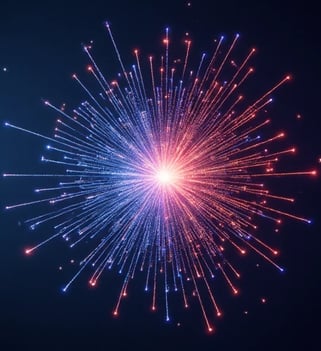